Misidentification of people based on ethnicity, gender, and age plagues the facial recognition industry, and it’s a continuing mission of ours to fix this problem.
From our 2012 inception, Kairos has licensed much of its core technology from other innovative AI vendors. By adding our own sizzle to it, we saw impressive adoption from businesses and developers, all around the world. However, it was always our vision to create our own.
Since joining Kairos earlier this year, my team and I have completely redesigned Kairos’ algorithmic system and architecture.
Today, Kairos face rec tech is 100% built in-house
Starting from scratch didn't come without its challenges, yet having the opportunity to design a new face recognition algorithm today was actually a blessing in disguise.
Over 6 years of doing business allowed us the time to witness the progression of deep learning techniques, the innovations of companies such as Apple (that little thing called ‘FaceID’), and society's reaction to an often divisive technology.
And, this year has really been the tipping point for the whole face recognition industry. Among the explosive growth, it’s also taken a bashing in the press; some of that criticism even coming from us— we all felt strongly about taking a stand against the misuse of the technology. And we all continue to feel strongly about this.
Melissa Doval, our interim CEO, told me what it means to her:
“As a first-generation daughter of Cuban immigrant parents, a woman and a minority—it’s my duty to continue to push Kairos to address the biases that exist in AI. Diversity is built into the Kairos DNA and our team will never abandon that mission.”
Powerful words. Which has struck me since I joined Kairos— everyone here cares deeply about how our technology impacts peoples lives. It’s always top of mind, and I love how we empower our employees, investors, partners, and customers to always be part of that conversation.
Now, we look forward with purpose
In this article I wanted to share some details about how we, at Kairos, are addressing the challenges of creating a truly diverse and inclusive facial recognition algorithm.
You'll notice some of our approaches are grounded in ‘well known’ machine learning practices, while others are far more experimental.
Combined I believe we have one of the strongest commercial strategies to tackling today’s AI biases; ensuring the future versions of our technology strive for the highest standards —it’s the right thing to do.
Beyond that, by sharing our own learnings and tactics, we hope we can inspire other face recognition companies looking to raise the bar.
How we deliver diversity & inclusivity in face rec:
- Diverse Data— We’ve undertaken the collection of the largest diverse database from all corners of the globe to ensure inclusivity. This database, curated by facial recognition experts, from different continents, will represent not just all ethnicities but different age groups and genders as well. When training our deep learning models we make sure to avoid any sampling bias by monitoring and tracking which data is used at each step of training, tuning, validation, and testing.
- Truly Diverse Data— We’re using a combination of real and synthetic facial data to achieve state-of-the-art performance. Utilizing research techniques such as GANs (Generative Adversarial Networks), we use real images to produce a larger number of synthetic images. For example; from 10,000 images collected manually we can generate 500,000 new facial images, introducing hitherto unknown variety in our training database. This ‘hybrid’ (real + synthetic) dataset is then further expanded by changing camera angles, backgrounds, lighting environments, and facial features using rendering face models.
- Algorithmic Accountability— We’re employing new and innovative measures to get to the underlying causes of bias in AI. We believe all AI should be transparent to everyone, not a ‘black box’. Our interpretability workflows can highlight why face recognition deep learning models make precise decisions. Meaning we now fully understand the bias that might occur and where we need to fix it.
Kairos and Untangle are pleased to announce a new partnership to help solve bias in AI.
Announcing our strategic partnership with Untangle
Much of how AI actually works is an unknown— as mentioned above, it’s a metaphorical ‘black box’. So, when it comes to assessing something like bias in AI, visibility on how the AI is ‘making decisions’ can be what makes or breaks an algorithmic model. Traditionally, this has been a very hard thing to assess; it’s not easy to ‘look inside the box’. Despite this, I knew it was critically important we had an objective way of to guarantee our AI was making good decisions.
Enter Untangle— the Singapore based startup that helps companies like Kairos understand and audit their AI tech, ensuring [deep learning] models are right for the right reasons. We’re thrilled to be working with them to help us keep our algorithms accountable.
This video shows the output 'facial-relevance map' as generated but the Untangle platform. As we can see, the person's face in the middle is not detected by the algorithm, the relevance map shows that the model doesn’t recognise his face to be a true face as the relevance scores are much lower. By extending this method to understand where in the network the facial features are not activating we can modify the network to generalize better to faces of all diversity.
“Partnering with Kairos is great for us at Untangle as they truly see the need to understand AIs and remove any inherent bias in their models. It’s companies like Kairos who will truly drive innovation and wider adoption of AI”— Jimmy Moore, CEO, Untangle
Untangle wants to see algorithmic accountability as the standard. They fundamentally believe that to have truly useful human to AI interactions—even AI to AI interactions— they need to be able to explain themselves in intuitive, understandable ways. For the general public to have trust in AIs they need to be auditable.
It’s clear Untangle plans to drive the change that needs to happen in the AI space, and we’re totally here for it.
—
In my next article I’ll be sharing the results of the work mentioned above, future goals for our algorithms, and give you a deeper dive into some of our in-house R&D efforts— stay tuned!
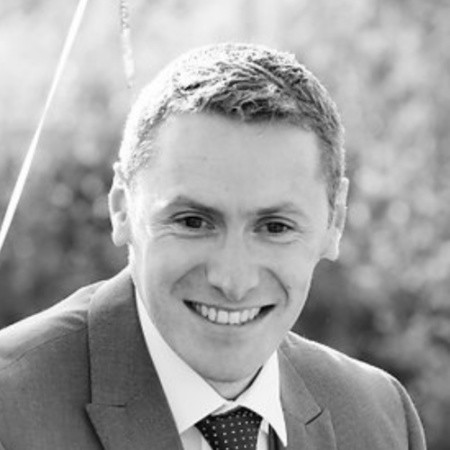
Dr. Stephen Moore
Stephen is the Chief Scientific Officer at Kairos— Serving Businesses with Face Recognition