One of the most talked-about topics in the field of facial recognition has been blink detection. There has been much scientific analysis done on blink detection over the last decade as scientists have discovered that there are a number of consumer and business uses for the detection of whether somebody is blinking or not.
What is Blink Detection?
Blink detection is actually the process of using computer vision to firstly detect a face, with eyes, and then using a video stream (or even a series of rapidly-taken still photos) to determine whether those eyes have blinked or not within a certain timeframe.
How Does It Work?
There have been quite a few studies undertaken on how to actually put blink detection into practice. In simple terms, the system needs to first determine whether a video (or series of pictures) contains a face. Once it has determined the presence of one or more faces it will then focus in on the eye features, and if they are occluded at any point it is assumed to be a blink.
Why is This Valuable?
There are a number of uses for blink detection. Probably the most common use, as far as consumers are concerned, has been in cameras and smartphones. The aim of this detection has been to help the photographer improve their photographs, by telling them when their subjects have blinked. The blink detection technology focuses on the eyes of people in the photograph (they can often work with up to twenty faces) and whenever a pair of eyes are occluded there will either be a message displayed on the lcd screen telling the photographer to delay taking their photograph, or the more advanced cameras are smart enough to simply snap the photo at a moment when all eyes are open.
This usage is rapidly becoming more common. Many mid to high end cameras come with it automatically built into the cameras. Blink detection has also been built into iOS7 and 8 (and there are apps in Android that also provide it). A number of apps related to photography use it. There is even an iOS app called BabySmile, which emphasises the fact that it takes photos using smile and blink detection. It ensures that in every baby's photo he or she has a smile on their face and their eyes are open.
A designer Mimi Zou has come up with an alternative photographic use for the technology in a concept called Iris, which uses blink detection in the camera in a reverse way. The blink detector is focussed on the photographer, rather than the subject, and releases the shutter when the photographer blinks. Widening or narrowing the eye controls the camera’s zoom. This idea has yet to reach production, and is still at the concept stage, but this is definitely an outside-the-box use for blink detection.
Another area where blink detection has been trialled in more laboratory-type situations has been measuring blinking of drivers, determining whether recording drivers’ blinking behavior can be used to spot drowsiness and inattentiveness before accidents happen.
Recognizing “Liveness”
Blink detection can also be used for “liveness” or “anti-spoofing” systems to see if a live person or a photograph is being verified using face recognition / detection. Blinking provides a signal which is easily detected and unique to faces. It is important to realise that we are talking about blinking here, not winking. With blinking you have two eyes moving together, and this is the key factor distinguishing blinking from other motions that may be happening. This ties in with the fact that eyes are symmetrically positioned with a fixed separation.
Blink detection is just one part of the analysis into microexpressions that can be aided by the analysis of still images and video. Because they happen so quickly, microexpressions can be difficult to recognize, but still images and video showing them can make them easier to perceive. Software involving frame rate manipulation allows the viewer to distinguish distinct emotions, as well as their stages and progressions, which are otherwise too subtle to identify. Blink detection is definitely part of this process.
What Can Stop Blink Detection from Working?
One of the most difficult tasks that any blink detection software has to overcome is the fact that our blinks can be very quick. Most blinks only last for 300 milliseconds (ms), approximately a third of a second. While it is theoretically possible to see blinks in a series of still photographs, it is just as likely that the subject will have blinked between shots, and it could have been missed completely.
Even when using video, the average blink only results in the eyes being completely closed for one to two frames of video. This makes live video analysis very difficult to do.
People are, of course, not robots. Everybody is different. Blinking is no exception, and there is quite some variability in blink rates from one person to the next. Adults blink on average at somewhere between four and 16 times per minute, whereas children and babies can blink as little as one to two times per minute.
Some of the camera manufacturers who introduced blink detection to their products in the technology’s infancy suffered from a few embarrassing teething errors. This 2010 Time article shows one such problem where some of the early cameras with blink detection were unable to distinguish blinking by people of some races.
There is still an industry-wide problem with liveness detection, that is wider than just blink detection, however. Someone who is very determined to defeat a facial recognition system could actually hold up an iPad with a video of a face blinking in front of the detector if they really wanted to fool the system. Indeed, scientists have analyzed that there are three main methods used to generate a face spoof attack: using a photograph of a valid user, reproducing a video of a valid user, and presenting a 3D reproduction of the face of a valid user.
A robust liveness detection system would need to provide tests for other things like detecting electronic devices, screens and other flat surfaces etc. Liveness detection is a problem that is not yet completely solved by the industry, although there is some promising research being done. The research come in two main areas. The first, static analysis, is based on the concept that during the manufacturing process of a photo attack there will be a certain loss of information and also peculiar noise will be introduced. The second focus of research is video analysis, that tries to detect, as humans do, facial physiological clues like blinks, mouth movements and changes in facial expression. Although, the research is still incomplete, it would appear that the best method of ensuring likeness is a mixture of both static and video analysis (including blink detection).
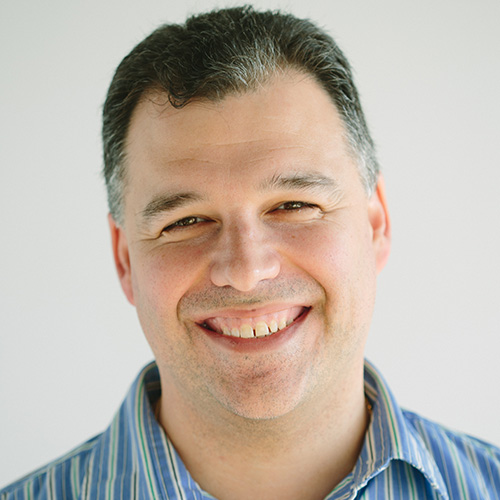
Cole Calistra
Cole is the CTO at Kairos, a Human Analytics startup that radically changes how companies understand people. He loves all things cloud and making great products come to life.